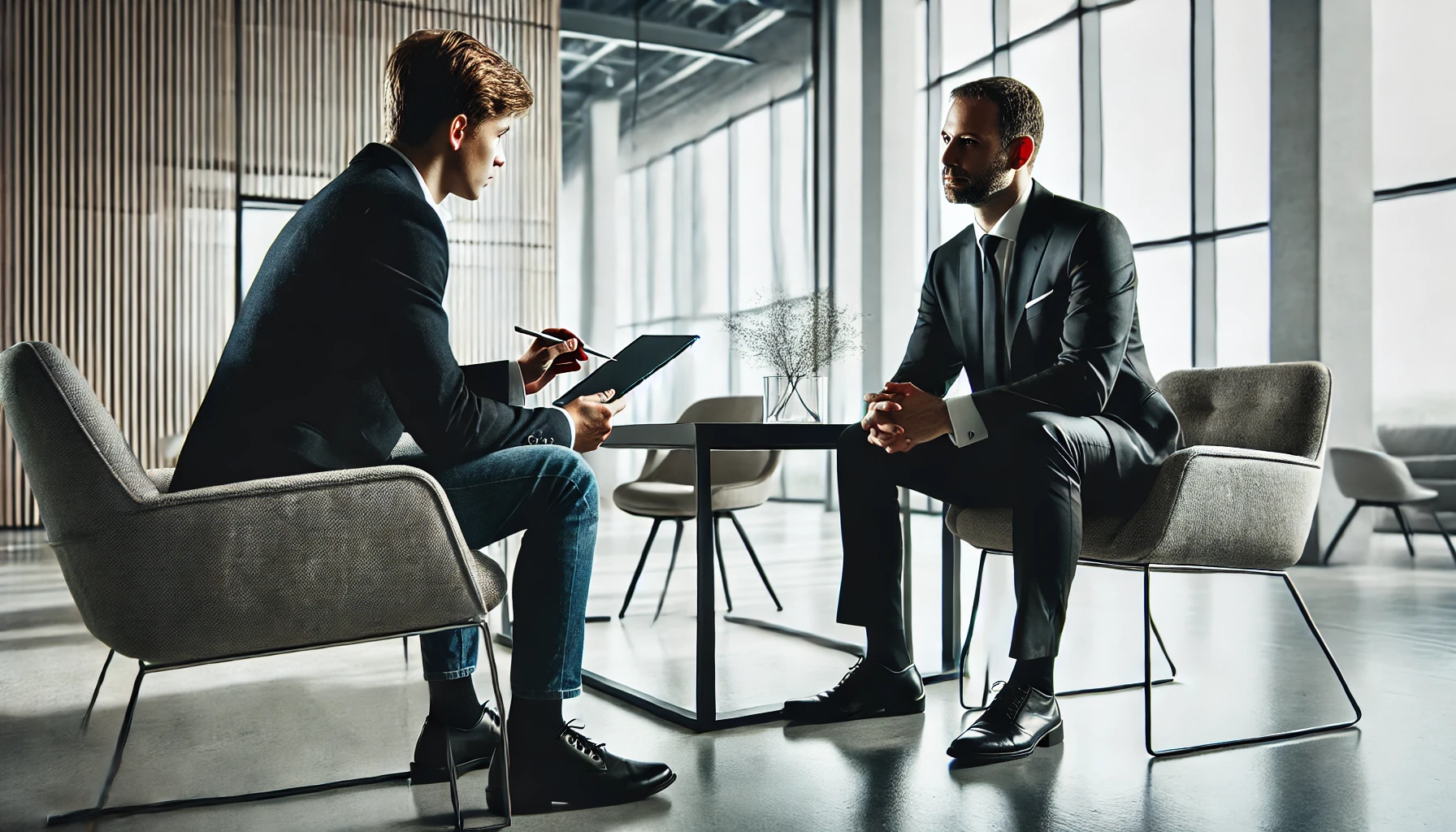
In today's competitive market, the role of C-suite leaders and boards of directors extends beyond traditional responsibilities to ensuring the company retains its top talent. With nearly one-third (30%) of American workers actively seeking new opportunities outside of the current company, according to the "State of Work Today" report, finding effective employee retention measures is more critical than ever. This trend is also evident among Canadian workers at 25% and European workers at 23%, making effective employee retention measures more critical than ever. Advancements in data analytics and machine learning offer powerful tools to predict and prevent employee turnover, and many companies already possess the necessary data.
Why This Matters: The High Cost of Employee Turnover
Employee turnover can significantly impact a company's bottom line. The costs associated with recruiting, onboarding, and training new employees are substantial, not to mention the potential loss in productivity and morale. By leveraging machine learning, C-suite leaders and boards of directors can gain valuable insights into which employees are likely to leave and take proactive steps to retain them.
The cost of employee turnover can be substantial, with even a single percentage point reduction in turnover saving a company between $350,000 and $1.5 million per 1,000 employees, depending on the difficulty of replacing those employees. Calculating the discounted net present value (NPV) over three years at a 10% discount rate, the savings range from approximately $870,000 to $3.7 million. These calculations highlight the significant financial impact that effective retention strategies can have, underscoring the importance of investing in measures to reduce employee turnover. Try this free cost of employer turnover calculator here.
Using AI for Predictive Insights
Artificial Intelligence (and machine learning in particular) taps into the extensive data sets maintained by human resources teams can build models to accurately predict and identify employees at risk of leaving. This not only helps in anticipating their behavior but also enhances management's understanding of why they might leave and what might motivate them to stay.
HR data sets typically include salary history, performance ratings, and disciplinary notes, along with information about internal job applications, managerial roles, critical talent status, and high-potential employee flags. These models can be customized to fit a specific company's data, providing insights based on the unique attributes of their workforce.
The concept isn't new—marketers have been using similar predictive models to retain customers in subscription-based businesses like streaming services and mobile phone providers. By analyzing customer data, these businesses can identify customers they may lose, discover actions to prevent them from leaving, and persuade others to buy more.
AI models effectively predicted which individual employees planned to leave within six months. For a large hospital struggling to retain nurses, machine learning helped accurately identify individuals at risk of quitting by examining multiple years of HRIS data. This allowed the hospital to suggest proactive measures to extend these nurses' tenures. By analyzing factors such as workload, shift patterns, and job satisfaction, the hospital could implement targeted interventions such as adjusting schedules and providing additional support.
Furthermore, the hospital adopted personalized communications aimed at addressing the specific concerns of nurses with a high flight risk. Tailored messages and regular check-ins helped management understand individual challenges and demonstrate a commitment to resolving them. In addition to this, personalized training and development programs were introduced, focusing on the career aspirations and skill gaps of at-risk nurses. By offering customized development paths and mentorship opportunities, the hospital not only retained valuable nursing staff but also fostered a more engaged and motivated workforce, ultimately enhancing patient care quality.
Optimizing Employee Preferences
Once a company identifies a high-performing employee who may be about to leave, the next step is to deploy relevant retention tools. The goal is to understand how sensitive workers are to specific changes in the value proposition represented by compensation, benefits, and other rewards.
Instead of directly asking employees what they want, which often results in impractical answers, well-designed surveys can compare the value employees perceive in different packages. For instance, a survey might reveal that boosting the monthly car allowance for top employees could make them perceive the value of that perk as almost double its cost.
More broadly, listening to employees through surveys makes it possible to analyze an array of benefits, ranging from health insurance coverage to retirement savings to vacation policy. The analysis is based on the benefit’s perceived worth as a fraction or a multiple of the actual expense. Conjoint analysis, which marketers use to understand the relative value customers place on product or service features, can help measure an individual’s sensitivity to changes in benefits and rewards.
Employee surveys make it easier to perform effective analyses of the best benefits options for employers and employees. Often, a total rewards package can be identified that 70% or 80% of employees think is better than their previous package but costs the employer thousands of dollars less per person per year. Using such tools, an employer planning on boosting spending on rewards and benefits can be confident it is doing so effectively.
Case Study: Predicting Employee Churn in Action
AI has shown efficacy in predicting employee turnover. For instance, a large federal government agency used machine learning to accurately identify individuals at risk of quitting or taking early retirement by examining multiple years of HRIS data. This allowed the agency to suggest proactive measures to extend these employees' tenures. Such predictive insights can be highly advantageous, providing organizations with a strategic edge in retaining valuable talent. The model's accuracy in forecasting high flight risk employees was impressive, often identifying at-risk individuals with over 90% precision. By leveraging AI, organizations can not only foresee potential turnover but also tailor their retention strategies to address specific issues, ultimately reducing costs associated with hiring and training new employees. Additionally, AI-driven analytics can uncover patterns and trends that might be overlooked by human analysis, leading to more informed and effective HR decisions.
By reducing their workload and shifting them into mentoring roles, some employees who would have retired early were retained, preserving their legacy and facilitating effective knowledge transfer to younger staff. Without this approach, these employees would have simply left.
Implementing Effective Retention Strategies
Recognize that in some instances, intervention may not be necessary. If an employee is a poor performer with a high flight risk, it may be more beneficial to let them leave rather than invest in retention efforts. The goal is to retain valuable employees and extend their tenure by addressing their specific needs and preferences effectively.
To effectively implement retention strategies, organizations must focus on several key areas:
Data-Driven Decision Making: Utilize HR data to inform decisions about employee retention, identifying patterns and trends that indicate potential turnover.
Customized Retention Plans: Develop personalized retention plans that address the specific needs and preferences of individual employees. For those at high flight risk, focus on delivering and communicating highly personalized value and highly relevant communication.
Enhanced Communication: Maintain open and transparent communication channels between management and employees to build trust and address concerns. Proactively engage with employees to understand their satisfaction levels and address any issues before they escalate.
Proactive Engagement: Regularly engage with employees to understand their satisfaction levels and address any issues proactively.
Personalized Professional Development: Provide opportunities for career advancement and tailored and individualized professional development plans to keep employees motivated and aligned with company goals.
Personalized Coaching: Understand the unique needs of employees with high flight risks, allowing managers to spend time addressing key concerns.
Personalized Total Rewards: Offer personalized, high-valued total rewards that are meticulously tailored to the unique needs and preferences of each employee. By ensuring these rewards are both generous and directly relevant to the individual, we enhance employee satisfaction, engagement, and retention.
Summary
C-suite leaders and boards of directors can leverage AI to enhance employee retention by utilizing advanced data analytics and machine learning tools. These technologies allow organizations to predict which employees are at risk of leaving and identify the underlying reasons for their dissatisfaction. By understanding these factors, companies can implement targeted interventions to retain top talent. This approach is not only cost-effective but also essential in today's competitive job market, where retaining skilled employees is crucial for maintaining productivity and morale.
The financial implications of employee turnover are significant, with costs associated with recruiting, onboarding, and training new employees. AI-driven insights enable companies to proactively address potential turnover, resulting in substantial savings. For example, reducing turnover by just one percentage point can save a company between $350,000 and $1.5 million per 1,000 employees. Over three years, these savings can range from approximately $870,000 to $3.7 million. These figures highlight the importance of investing in AI-driven retention strategies.
AI models, using HR data such as salary history, performance ratings, and job satisfaction, can accurately predict employees at risk of leaving. Organizations can then tailor retention efforts, such as adjusting schedules, providing additional support, and offering personalized development programs, to meet the specific needs of these employees. This targeted approach not only helps retain valuable staff but also fosters a more engaged and motivated workforce. By leveraging AI, C-suite leaders and boards can ensure effective knowledge transfer, preserve institutional legacy, and maintain a competitive edge in talent retention.
To explore methods for predicting employee flight risks and enhancing retention, find innovative solutions customized to your organization's specific needs.